Exploring AI Agents with OpenAI Swarm

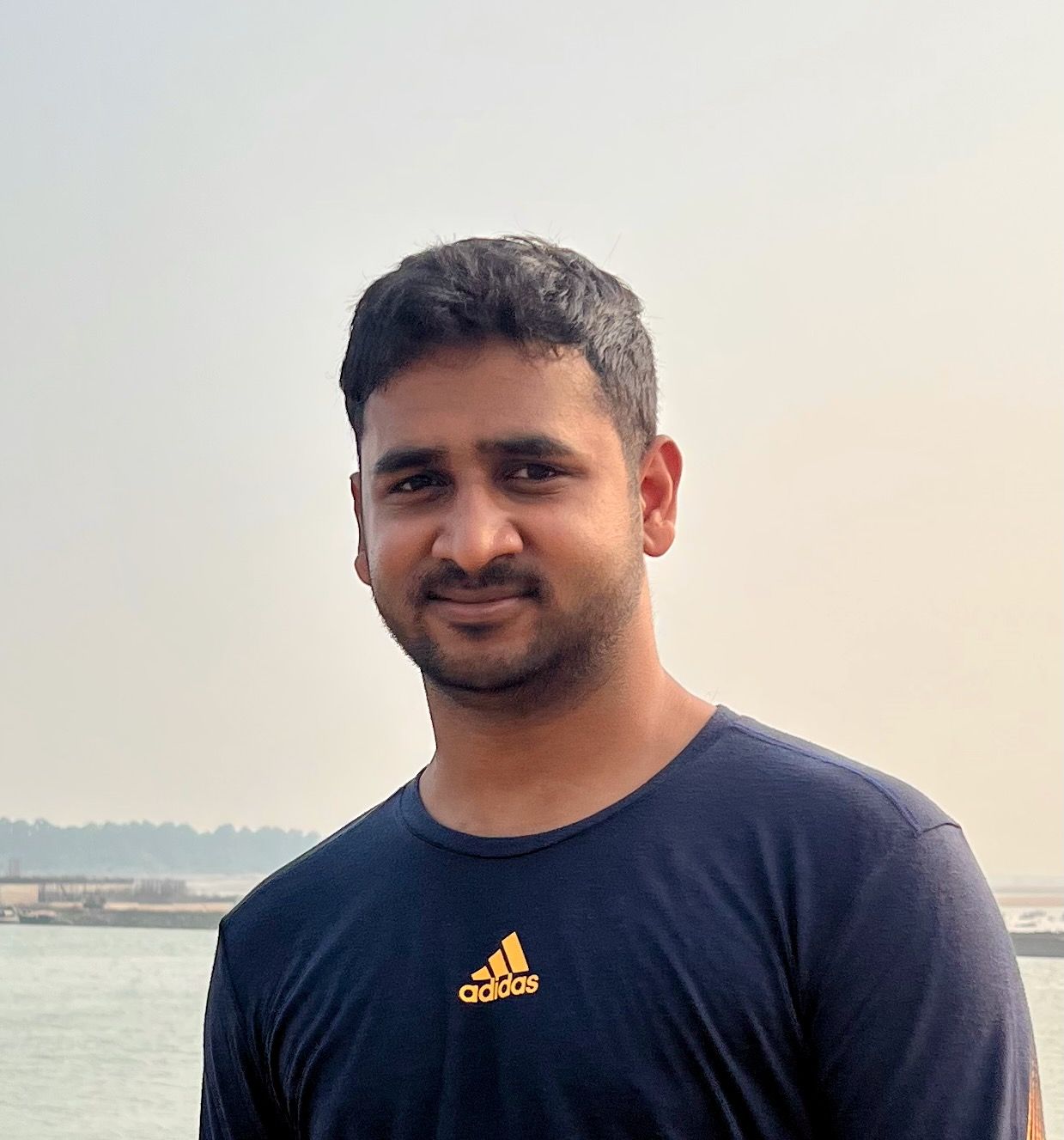
OpenAI’s Swarm Framework is an innovative approach to multi-agent collaboration that leverages principles of swarm intelligence to solve complex problems. Inspired by the collective behavior observed in nature—from flocks of birds to schools of fish—this framework is designed to coordinate a large number of intelligent agents working in tandem, allowing them to share insights and converge on high-quality solutions.
Introduction
Traditional AI systems often rely on a single agent or model to tackle a problem. However, many real-world challenges—such as dynamic decision-making, complex optimization, or large-scale problem-solving—benefit from a decentralized, multi-agent approach. OpenAI’s Swarm Framework addresses these challenges by enabling multiple agents to interact and learn from one another, resulting in a system that is more robust, adaptive, and capable of tackling tasks that are too complex for individual agents.
What Is Swarm Intelligence?
Swarm intelligence is a field of study that examines how simple agents following basic rules can produce collective behaviors far more complex than the sum of their parts. In the context of AI, this means that a group of agents can:
• Explore diverse solutions simultaneously: Each agent can approach the problem from a different angle.
• Share findings in real time: Communication between agents leads to faster convergence on optimal solutions.
• Adapt dynamically: The collective can respond to changes in the environment without central control.
Architecture of the Swarm Framework
The Swarm Framework is built on a decentralized architecture that facilitates seamless communication and coordination among agents. Key components include:
1. Agent Nodes
Each node in the swarm acts as an autonomous agent equipped with its own processing capabilities and decision-making logic. These agents can be based on various OpenAI models or custom implementations tailored to specific tasks.
2. Communication Layer
A robust messaging system allows agents to exchange data, hypotheses, and feedback in real time. This layer ensures that insights gathered by one agent are quickly disseminated across the swarm.
3. Coordination Mechanisms
Rather than relying on a single central controller, the framework uses consensus algorithms and dynamic leader election techniques to coordinate collective decision-making. This decentralized approach enhances fault tolerance and scalability.
4. Aggregation & Synthesis Module
Once agents have explored different facets of a problem, their individual outputs are aggregated. This module synthesizes diverse perspectives into a coherent, high-quality solution—often outperforming what any single agent could achieve on its own.
Technical Details and Use Cases
Technical Highlights
• Asynchronous Operations: The framework supports asynchronous communication, allowing agents to operate independently while still contributing to the collective goal.
• Adaptive Learning: Agents continually update their strategies based on feedback from peers, leading to emergent behaviors and improved performance over time.
• Scalability: The decentralized design means that additional agents can be integrated seamlessly, making it ideal for scaling up to very large problem domains.
Potential Use Cases
• Complex Problem Solving: For optimization tasks, such as logistics or network design, where multiple variables interact in non-linear ways.
• Autonomous Robotics: Coordinating fleets of drones or robots to explore, monitor, or interact with environments in a cooperative manner.
• Distributed Research: Accelerating scientific discovery by allowing diverse AI agents to analyze large datasets and collaborate on generating hypotheses.
Challenges and Future Directions
While the promise of the Swarm Framework is significant, it also introduces challenges:
• Synchronization Overhead: Ensuring smooth communication among a large number of agents can be complex.
• Robustness to Noise: The system must filter out inconsistent or irrelevant information to prevent degradation in performance.
• Real-Time Adaptation: Balancing exploration and exploitation in dynamic environments remains an active area of research.
Looking ahead, enhancements in network protocols, more efficient consensus algorithms, and deeper integration with other AI modalities could further expand the capabilities of the Swarm Framework.
Conclusion
OpenAI’s Swarm Framework represents a bold step forward in multi-agent AI systems. By harnessing the power of collective intelligence, it opens up new possibilities for solving problems that require diverse perspectives and rapid, adaptive decision-making. Whether for complex optimization, autonomous robotics, or distributed research, this framework is paving the way for the next generation of AI solutions.