Comprehensive Guide on Vector Databases

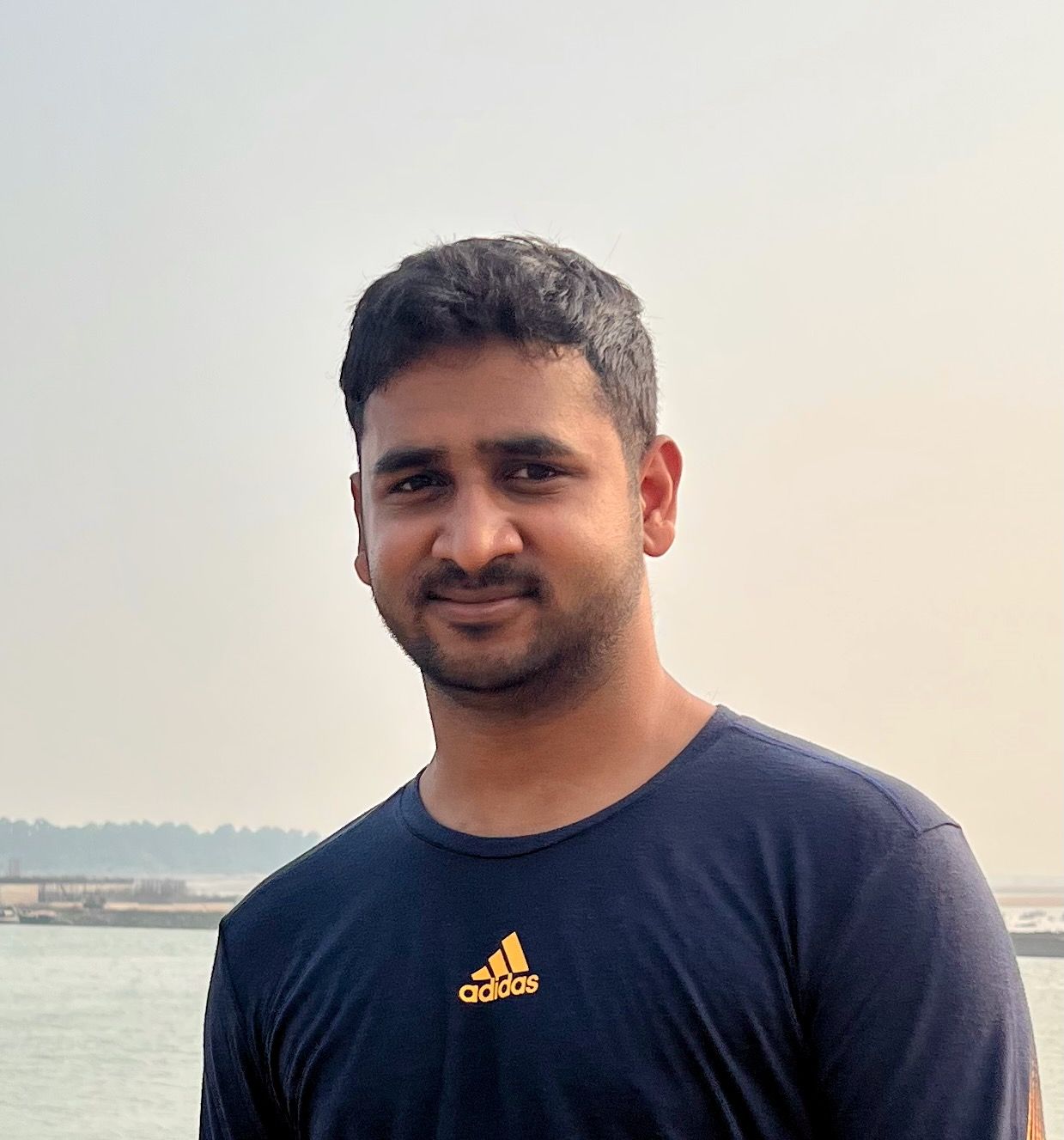
Comprehensive Guide on Vector Databases
Vector databases have emerged as a powerful tool for storing and querying high-dimensional data, especially in the fields of artificial intelligence and machine learning. This guide will walk you through the basics, key concepts, and best practices for working with vector databases.
Introduction
In today’s data-driven world, traditional relational databases often struggle to handle the unique requirements of AI applications. Whether you’re building recommendation systems, semantic search engines, or other AI-driven solutions, vector databases offer a specialized solution to store, index, and query complex, high-dimensional data like embeddings. This guide provides a comprehensive overview of what vector databases are, why they matter, and how to integrate them into your projects.
What Are Vector Databases?
Vector databases are designed to manage and process vector data—numerical representations of data points that capture the semantic meaning of items such as text, images, or other content. Unlike conventional databases, vector databases are optimized for:
• Similarity Search: Quickly finding items that are semantically close based on distance metrics.
• High-Dimensional Data Handling: Efficiently storing data in spaces that can have hundreds or thousands of dimensions.
• Real-Time Querying: Delivering fast, approximate nearest-neighbor (ANN) searches crucial for real-time applications.
Why Use Vector Databases?
The rapid evolution of AI and machine learning has led to an explosion in the use of embeddings. Here’s why vector databases are essential:
• Scalability: Manage millions of high-dimensional vectors without compromising performance.
• Efficiency: Use specialized indexing techniques (e.g., HNSW, IVF, PQ) to quickly perform similarity searches.
• Versatility: Integrate with various AI frameworks to power applications like recommendation systems, image search, and natural language processing (NLP).
Key Concepts and Techniques
Understanding a few foundational concepts is crucial:
1. Embeddings: These are fixed-length numerical representations of data items. They capture semantic information, allowing similar items to be close in vector space.
2. Distance Metrics: Common metrics like Euclidean, cosine similarity, or Manhattan distance help determine how “close” or similar two vectors are.
3. Indexing Methods: Techniques such as Hierarchical Navigable Small World (HNSW) graphs or Inverted File (IVF) indexes improve query speed by narrowing down search areas.
4. Approximate Nearest Neighbor (ANN): These algorithms balance speed and accuracy, enabling rapid retrieval in large datasets.
Popular Vector Databases
Several vector database platforms have become popular among developers and data scientists:
• Milvus: An open-source vector database known for its high performance and scalability.
• Weaviate: Offers built-in machine learning models, allowing for semantic search and real-time updates.
• Pinecone: A managed service designed for ease of use, with robust features for large-scale vector operations.
Each of these options has unique strengths and may be chosen based on the specific needs of your application.
Getting Started: A Practical Walkthrough
Step 1: Setup and Installation
Most vector databases offer comprehensive documentation for installation. For example, if you’re using Milvus, start by installing the Docker version or using a cloud-hosted service. For Weaviate or Pinecone, follow the provider’s setup guides to connect your environment.
Step 2: Indexing Your Data
Once installed, you need to convert your data into vectors. This can be done using popular ML models (e.g., BERT for text or ResNet for images). After obtaining embeddings, load them into your vector database. Use the platform’s API or SDK to create indexes that optimize similarity searches.
Step 3: Querying the Database
Perform searches by calculating the distance between the query vector and stored vectors. Use the built-in ANN algorithms to retrieve the most similar items efficiently. Fine-tune parameters such as search depth and approximation levels to balance speed and accuracy.
Best Practices for Optimal Performance
• Data Normalization: Ensure that your vectors are normalized if required by the distance metric.
• Regular Index Updates: Keep your indexes up-to-date, especially in dynamic environments where data is constantly changing.
• Monitor Performance: Use monitoring tools to track query performance and adjust configuration settings as needed.
• Experiment with Parameters: Fine-tune search parameters and indexing techniques based on the specific characteristics of your dataset.
Real-World Use Cases
Vector databases have broad applications, including:
• Recommendation Systems: Enhancing product or content recommendations by finding similar items.
• Semantic Search: Improving search results by understanding the meaning behind queries.
• Image and Video Retrieval: Locating similar images or video frames based on visual content.
• NLP Applications: Powering chatbots and virtual assistants with contextual understanding.
Future Trends
As AI continues to evolve, vector databases will play an increasingly important role in processing and retrieving high-dimensional data. Innovations in indexing algorithms and integration with emerging AI models promise to make these databases even more powerful and efficient.
Conclusion
Vector databases represent a critical component in the modern AI ecosystem. They offer the scalability, speed, and efficiency required to handle the vast amounts of data generated by machine learning models. By understanding the fundamentals and best practices outlined in this guide, you’ll be well-equipped to leverage vector databases in your own AI projects.
Stay tuned for more in-depth tutorials and case studies to help you maximize the potential of vector databases.